|
Delineating marine microbial taxa |
This dataset is provided as supplementary material to the following article:
Louca, S. (2024). Machine learning models for delineating marine microbial taxa. in review
Overview
The role of changes in gene content in the divergence of major microbial taxa is poorly understood, and reciprocally, our ability to delineate higher microbial taxa such as phyla or orders based on genomic content is limited.
Addressing these gaps is important for biodiversity assessments, the discovery of novel taxa and functions and for macroevolutionary theory.
In this study, we develop machine learning models, based on a multitude of genome similarity metrics, to determine whether any two marine bacterial and archaeal (prokaryotic) metagenome-assembled genomes (MAGs) belong to the same taxon, from genus up to phylum level.
Considered metrics include average amino acid and nucleotide identities, as well as the fraction of shared genes within various gene categories, applied to a dataset of 26,466 previously published MAGs (14390 species-level bins) from 106 surveys worldwide.
At all taxonomic levels, the balanced accuracy of models exceeded 92%, suggesting that simple genome similarity metrics can serve as good taxon differentiators.
Predictor selection and sensitivity analyses revealed gene categories particularly correlated to taxon divergence.
Predicted taxon delineations were further used as inputs to clustering algorithms, in order to de-novo enumerate marine prokaryotic taxa in the data.
Accumulation curves and global richness estimates based on those taxon enumerations suggest that more than half of extant marine prokaryotic phyla, classes and orders, and more than one fifth of genera, have already been sampled by genome-resolved metagenomic surveys.
The eventual recovery of MAGs representing most marine prokaryotic genera thus appears to be a realistic target within reach.
Downloads
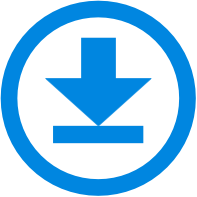 | Overview of metagenomic studies from which genomes were obtained. |
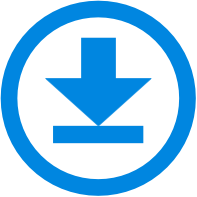 | Overview and accession numbers of MAGs. |
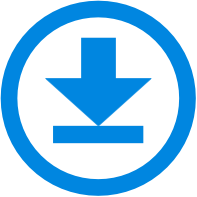 | Presences/absences of KOfams (KEGG orthologs) in each SGB. |
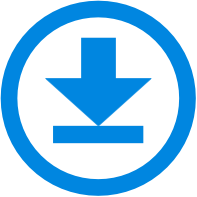 | Fitted taxon ingroup/outgroup classifiers (pickles of scikit-learn classifiers). |
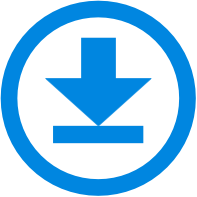 | Python code demonstrating the greedy clustering algorithms discussed in the paper. |
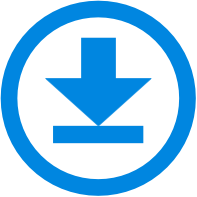 | Python script for classifying units (e.g., genomes) into ingroup/outgroup and clustering units into groups (e.g., taxa). |
|
|
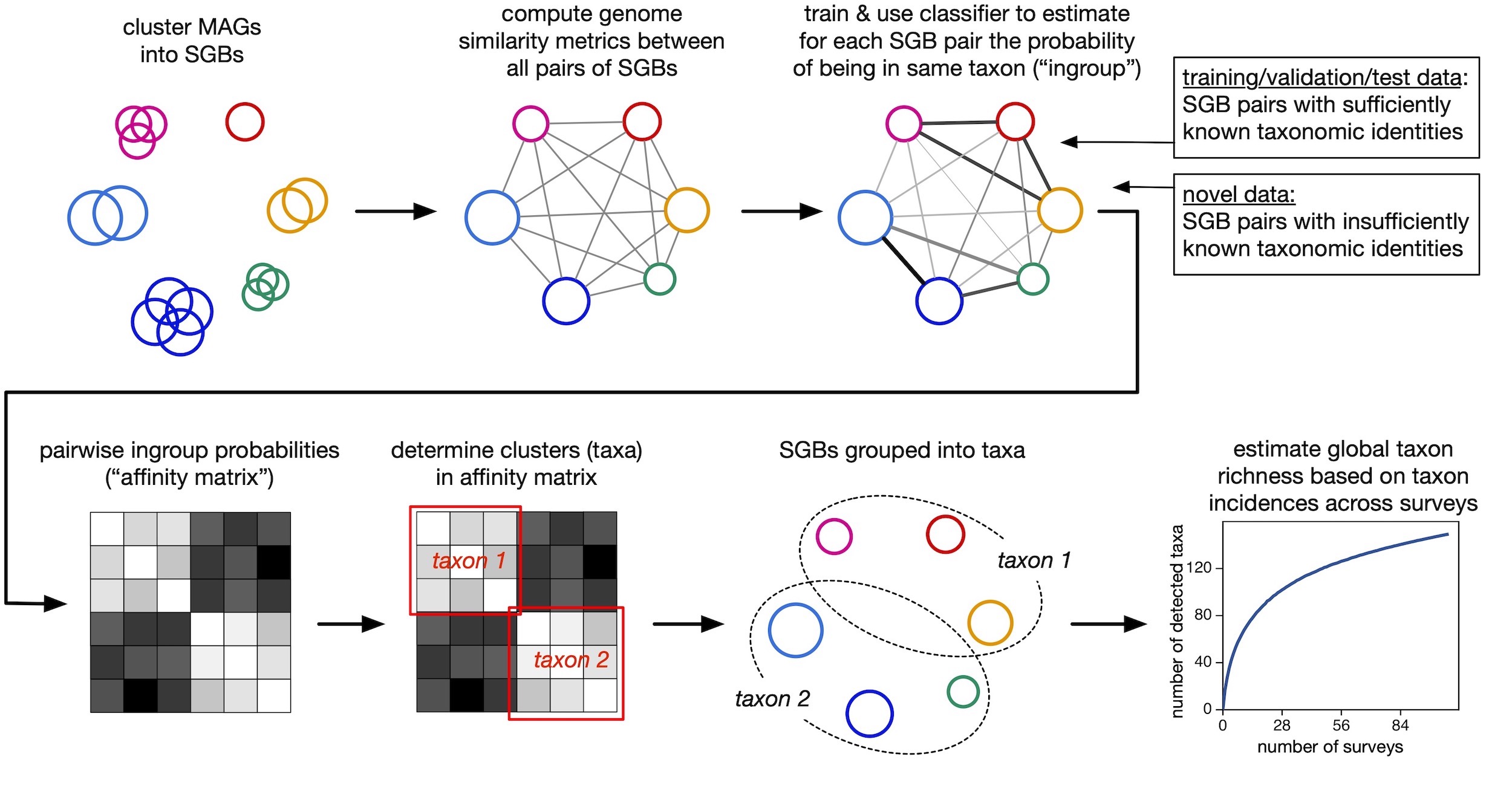 ×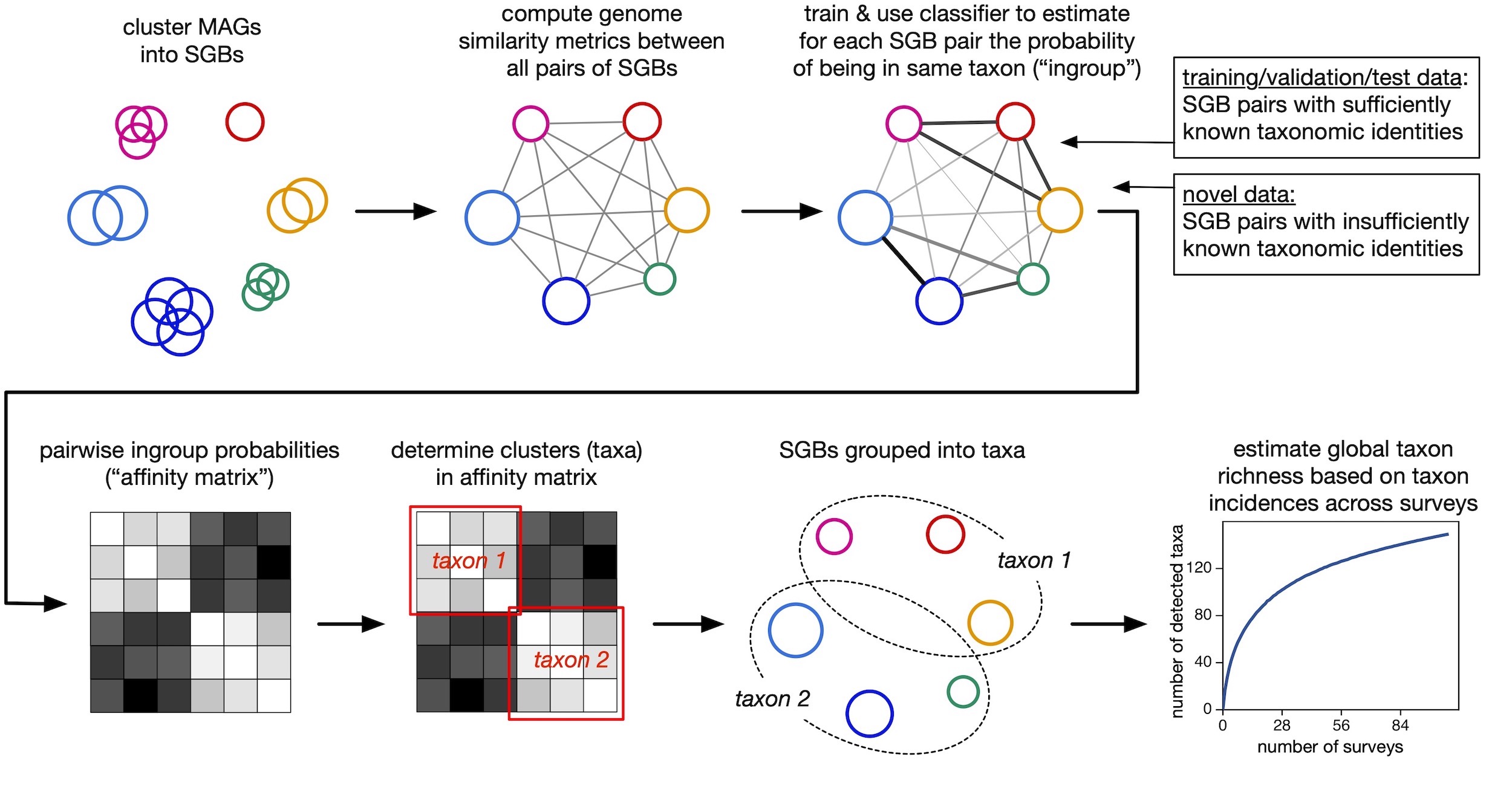
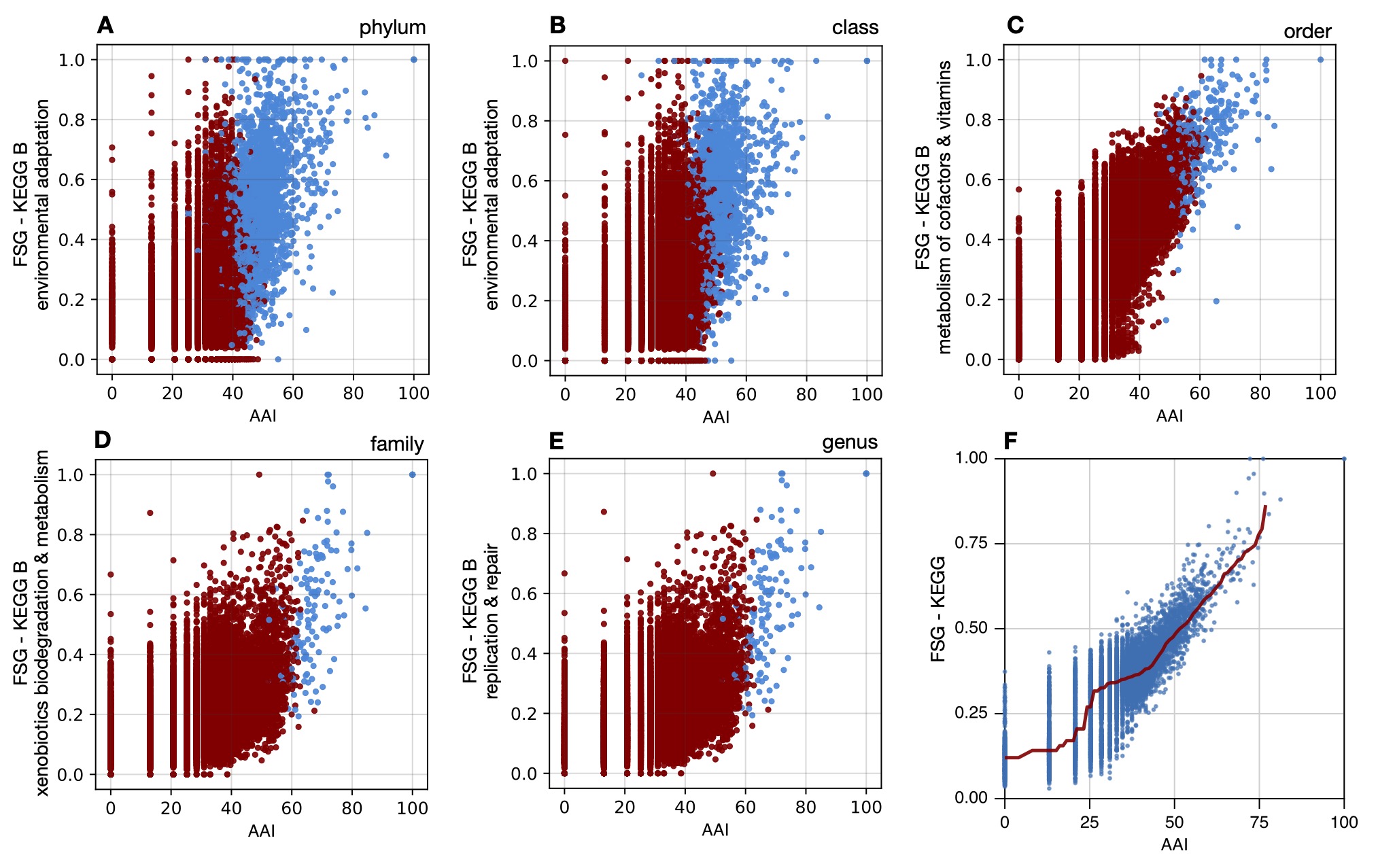 ×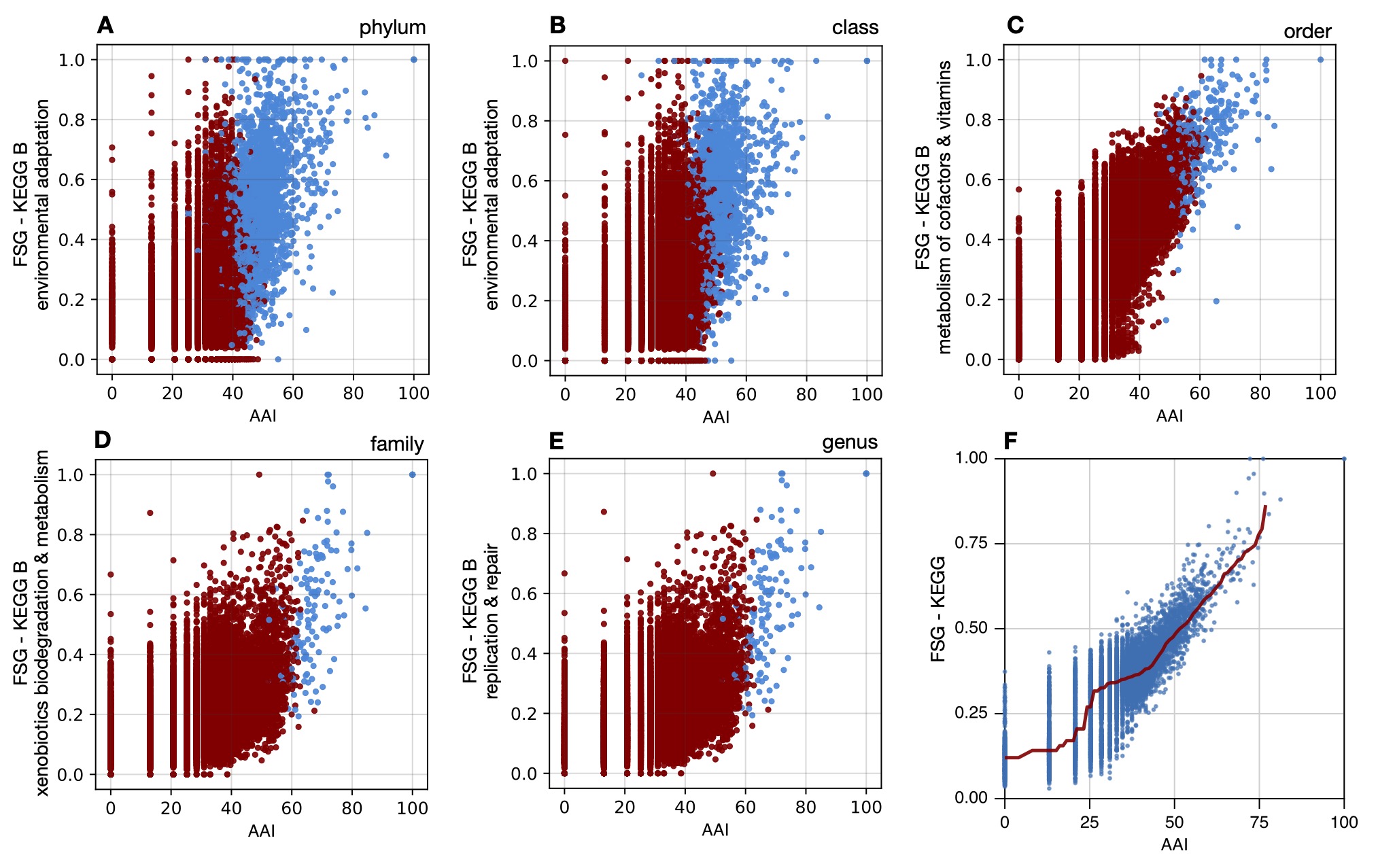
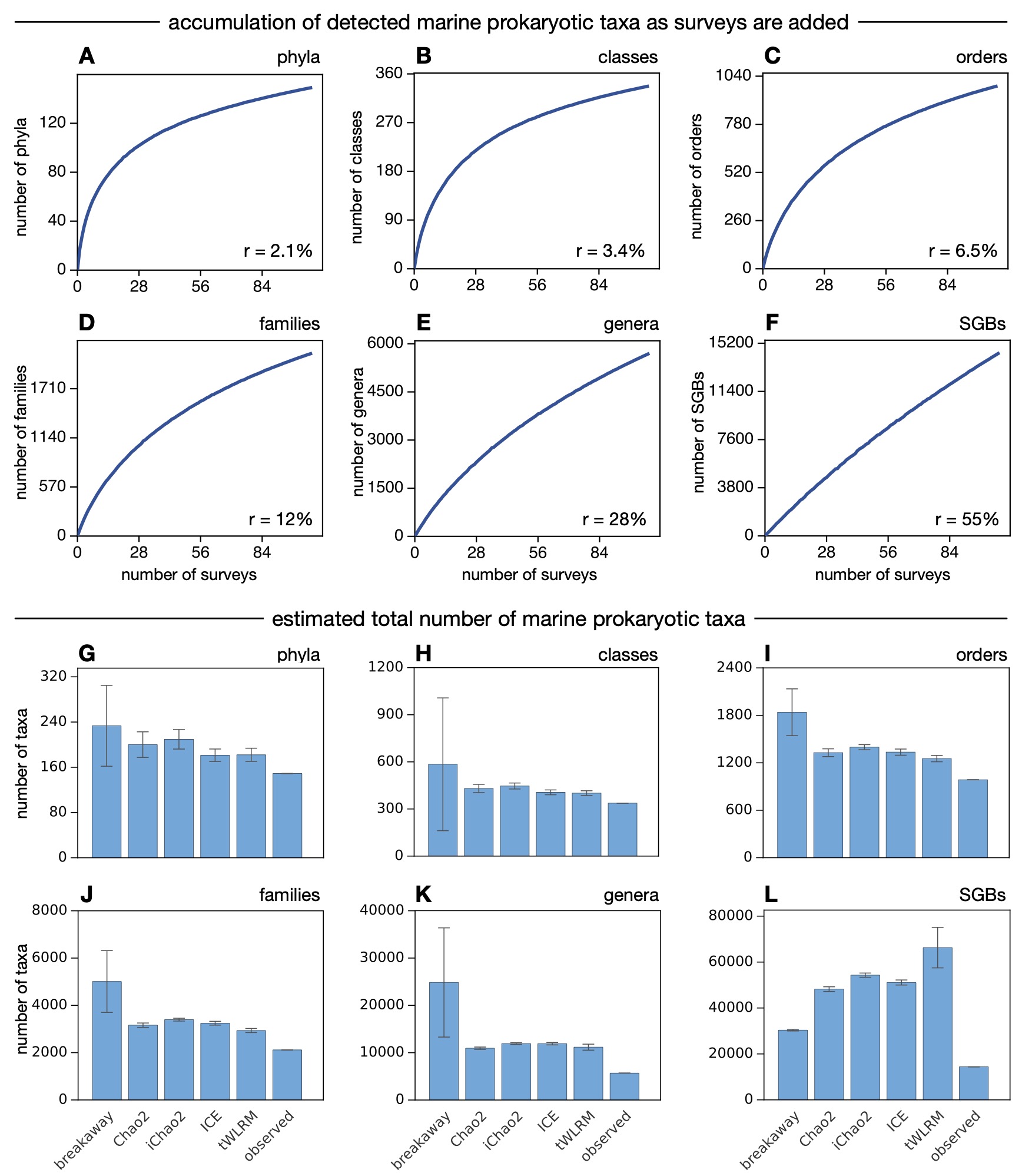 ×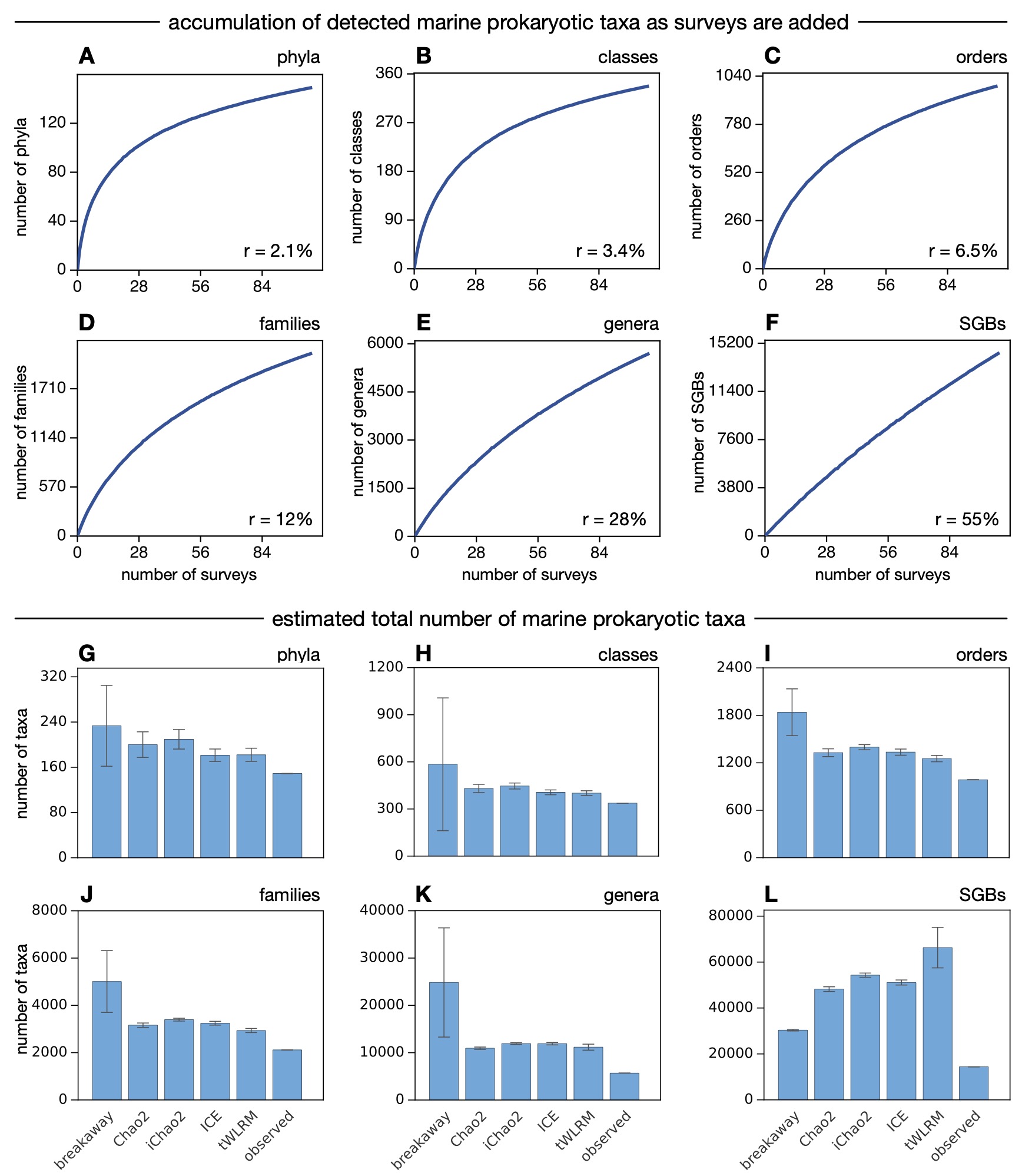
|
|
|
Louca lab. Department of Biology, University of Oregon, Eugene, USA © 2025 Stilianos Louca all rights reserved
|
|
|