Chapter 1 Initial conditions
Metrics is marketing
Throughout our time together we will treat data and inference with an eye for the market in which these tools and results exchange. We’re often sellers in this market, though we want to be informed in both “seller” and “buyer” roles.
Evaluate the following…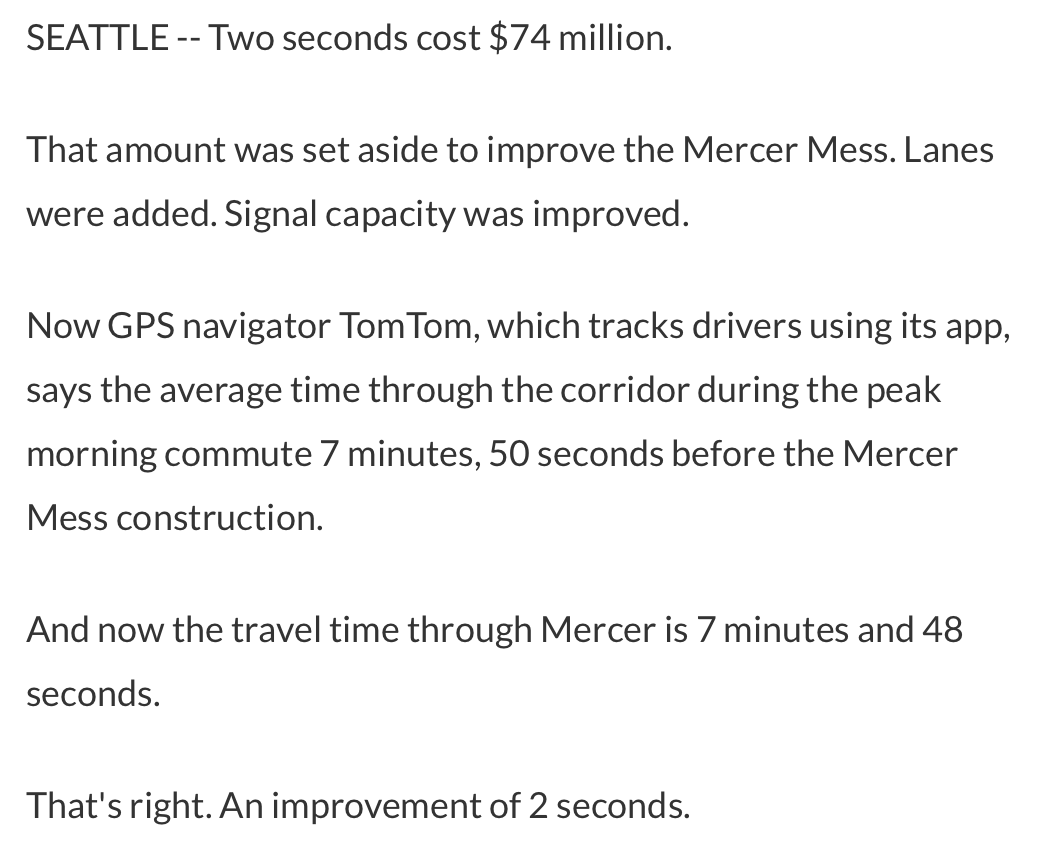
Figure 1.1: Fig: KOMO News, 16 Oct 2018
And this…
Was this “a colossal waste of taxpayer money?”
- Wait… we’re economists.
- Did prices change? Did behaviors respond to these prices?
- If each Seattle commuter was assigned to a specific mode/route, then the $74 million doesn’t seem to have served the commuters along the “Mercer Mess” route very well at all. That’s true.
- Don’t we anticipate a behavioral response to the $74 million in improvements, though?
What prices changed with these improvements?
- What is a price? The ratio at which two things exchange.
- The price of commuting by car fell ⇒ more people commute by car
- The price of that specific route fell ⇒ more people take that route
The Mercer corridor now handles 30,000 more cars a day than it did before the improvement project, without any increase in travel time.
The headline could have read “Seattle road improvements allow 10 million extra trips per year with no increase in travel times.”
Be savvy… be clever… have healthy skepticism, and an eye for the role of marketing in inference statements.
Know yourself
The best science comes from people who know themselves
How do you form your empirical priors?
- Experience?
- Institutional knowledge?
- Theory?
For example, how would you model economic agents in their pursuit of science, or profit, or pet project?
- You’re not suspending that they would maximize utility when you consider the players in this game, are you?
So, what is your empirical prior?
Is your prior that the statistics are sound? Well identified?
- More important… is your prior that the inference is sound?
Do you tend to believe the sign, but not the magnitude?
- Where does your confidence come from?
My objectives include challenging your priors
If your prior is to believe the magnitudes of relationships, I would like to challenge you to consider the ways in which this might be a stretch.
If you tend to believe the signs of relationships, I would like to challenge you to consider the ways in which even this might be a stretch.
If you think it’s all meaningless, or that “statistics can prove anything,” I would like to challenge this prior. (They can’t.)
(In fact, we prove nothing, really. We assume a lot of stuff, though.)
The bottom line…
We need justifiable confidence.
- When we’re right, to be confidently right.
- When we don’t know if we’re right… be confident about what it is we don’t know.
- When “they” don’t know either, and we know they don’t (or can’t)… to be confident about that, too.
Healthy skepticism
I have never been so convinced of the need for us to have healthy skepticism… skepticism that restricts inference from both directions.
- Most often, it will be in constraining the limits of what people are attempting to sell, or pass off as efficacious. However, we must also recognize that in light of the fundamental econometric problem, causal inference is established by assumption, and there are articulable conditions under which any of the results we establish are “correct.” We might not believe those assumptions, but the logic running from assumption to inference can still be established and defended.
Never believing a result belies a shaky understanding of the role of data. Likewise, to collapse on some notion that you can say anything with data is immature. Be mindful of both Type I and Type II errors, essentially.
Sometimes, it’s just about asking good questions. Evaluate the following, for example.
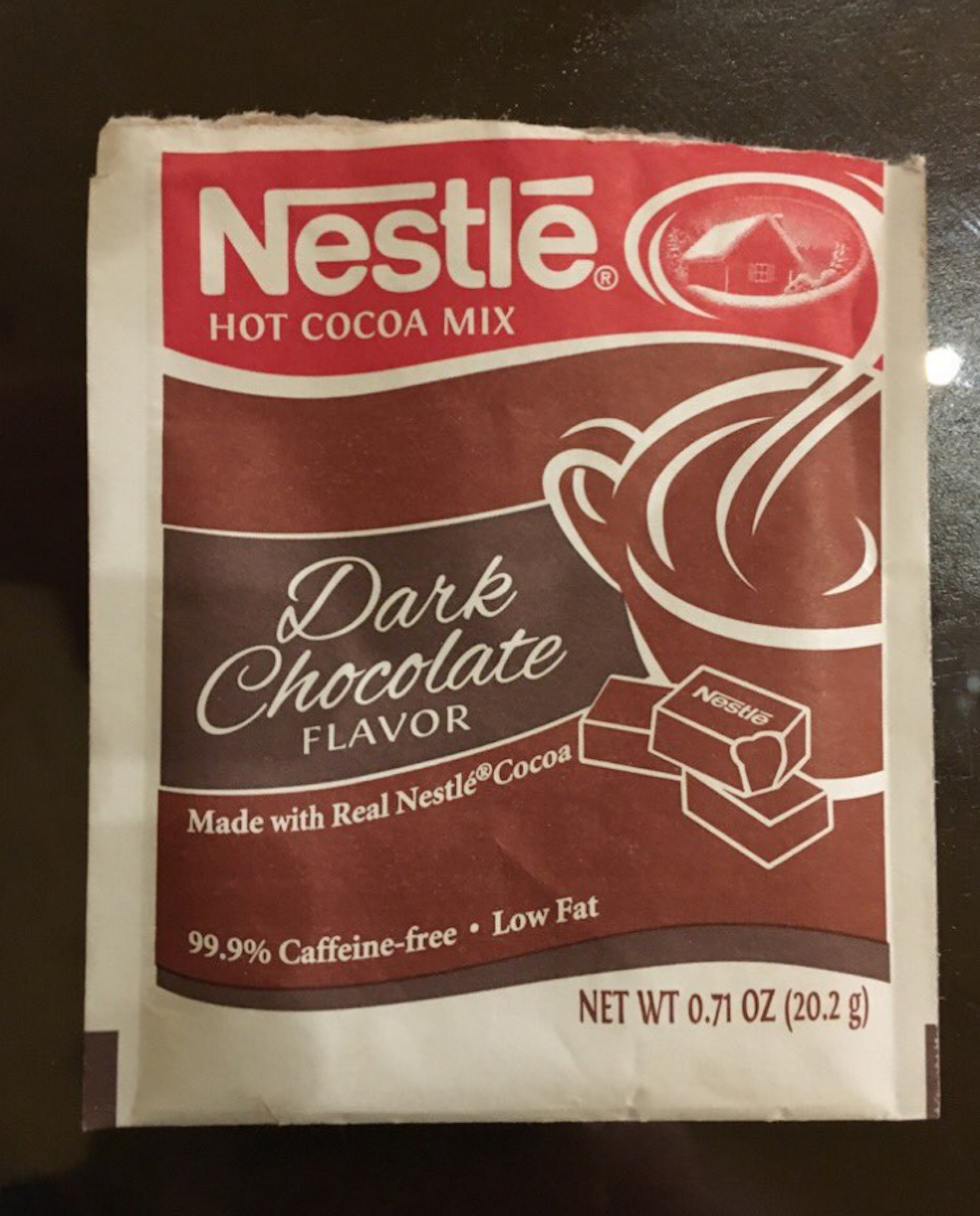
Figure 1.2: Be inquiring enough to ask… “99.9% of what?”
There are 249 milligrams of caffeine in the average 12oz Starbucks coffee.
- About 20.75 mg of caffeine per ounce… 0.02075 grams
- An ounce of water weighs about 28 grams
- A Starbucks drip coffee is about 0.074% caffeine by weight
Yes… coffee is 99.9% caffeine free!
Course objectives
In my mind, the purpose of this course is twofold.
1. We will reflect again on the philosophy of science, and the intersection of theory and empirics.
- To that end, we will learn data management and related skills that allow us to better focus our efforts on testing interesting and/or important economic theory – extracting relevant information from data that advances knowledge in a variety of areas.
2. We will develop the techniques of causal inference now common to modern applied-microeconomic research.
- Our self interest should demand that we follow the advance of causal inference. Moreover, we will want to be able to engage in the intersection of those techniques and research questions or economic issues, and thereby mature in our understanding of the discipline.
If there’s magic available, it’s somewhere close to the intersection of data and ideas.
- In the end, you should find yourself first with research questions, and then toolkits at the ready to inquire into those questions. In a world that is quickly embracing data-driven decisions, I’m encouraged. However, opportunities for sloppy inference are also abundant.
Logistics
We will meet from 1200p-1350p on each Monday and Wednesday of the term.
Software
You are expected to have your own copy of Stata or R or Python or a suitable substitute.
If they were zero cost, you would clearly learn them all. I’m twenty years into iterations of “Oh, this will be what everyone uses.” Jan ’19 update… Fed-bank job wants stata, consulting company wants R and SAS, litigation-support will still tend to want SAS, Apple wants R/Python and SQL (and more-specialized stuff that you signal your ability to acquire by having R/Python and SQL).
The bottom line has always been to get the job done. And… “the job” is still (I believe) more about ideas and knowledge aquisition than it is about coding language. I think you should adopt something that works, learn all of them as you are lead to, and be an evangelist only of sound, clever inference, and efficient analysis.
Grades
Hands-on experience with real data remains the best way to develop practical expertise. Thus, I plan to continue with the tradition of requiring an empirical project or suitable proposal from all students taking this course for credit. I recognize that a ten-week quarter does not typically provide one enough time to work through all of the different phases of a project at one’s leisure. That said, I do expect those who are appropriately engaged in the course to benefit from the experience.
With regard to the paper
Your first task is to identify a research question and identification strategy that you can realistically expect to have in hand and ready to go (by the end of the third week of the quarter?). Ideally, this will be part of your ongoing research agenda and the work you put into it this term will be evidenced in your written work down the road.I acknowledge that ten weeks is an absurdly short time-frame for completing publishable-quality empirical work. It is quite ample, however, to take steps toward publishable-quality empirical work. A replication exercise may be a reasonable substitute for the paper. See me if this option appeals to you.
Assignments
If/when I issue assignments, please follow instructions carefully. I am wanting to approach the assignments with some flexibility — I even have in mind that those who have active research agendas and discuss them in our times together might benefit from including aspects of their original empirical work as assignments in the class.
The final exam
We may have a written exam, an oral exam, or take home… all TBD.
Rubric
Your overall grade will be contributed to by the following:
- 20% Participation
- 30% Assignments
- 20% Exam
- 30% Paper
I reserve the right to extend “revise and resubmit” responses to any assignment you have submitted. I will do this when I believe there are sufficient benefits to you re-doing a given assignment, so ask that you do not interpret these outcomes as punitive.